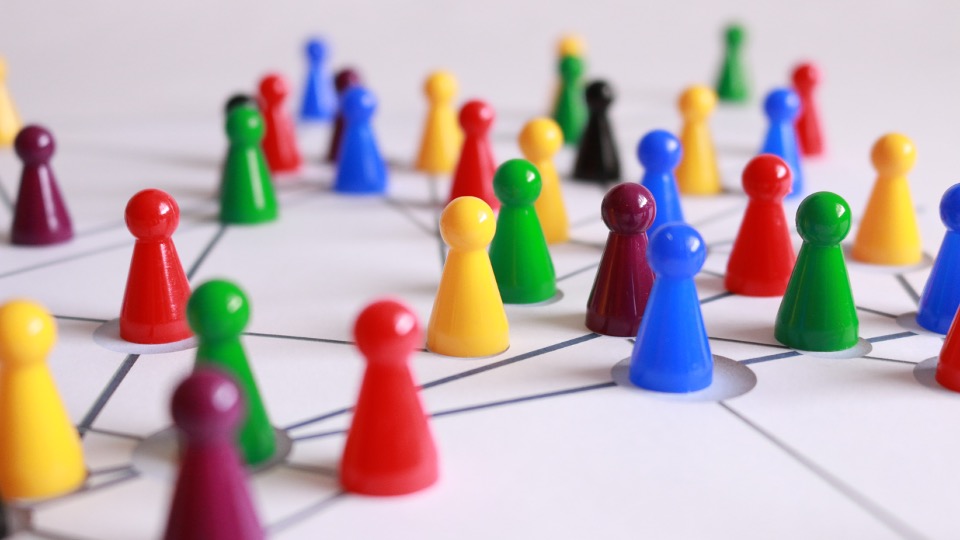
Comparisons, the saying goes, are odious. But they are the only way of learning from data. Confronted with data on several different units of observation (whether retail outlets, oil rigs, factories, customer service centres, or whatever), we naturally start to make league tables along various performance metrics or health indicators, with the hope that the poor performers can learn from the best performers.
The problem is that the manager of a unit in the worst-performing quarter of the database will immediately identify ten reasons why his unit is different from the best performers, along dimensions he cannot change. There will often be no observation exactly like his on all ten dimensions.
A common remedy for this is to cluster or categorize observations along each dimension (e.g. “large” vs “medium” vs “small”; or “urban” vs “suburban” vs “rural”; or “north” vs “midlands” vs “south”), and compare within cells of the resulting matrix. The problem with this approach is twofold:
- If you enumerate just 3 categories along 10 different dimensions, you get 310 cells (=59049), so you need a database of hundreds of thousands of observations to have several in each cell and so gain any insights. You will also be throwing away 99.998% of the sample as being irrelevant, which cannot really be the case.
- Some observations will be firmly in the middle of a category, but many will be borderline and so you will get heated debate about where exactly to draw the border.
PIMS has developed two methods over the years to solve this problem. The first step is to do research on the database using cross-tabulations and common sense to identify which dimensions of difference actually make a difference to performance. This naturally leads to multivariate statistical modelling using regression or similar methods to quantify which are the really powerful performance drivers, and enables a comparison of actual performance against “par performance” derived from the position of a this unit on the key drivers. The performance impact of each driver can be calculated as the effect of moving from the database average to the actual value of this observation on the driver, taking the drivers “most beyond management control” first. “PIMS method one” is to use the model directly to see where to position the observation on the key controllable drivers.
“PIMS method two” is to find look-alikes. This proceeds by calculating a distance from this observation to each other observation in the database along the drivers beyond management control (there are various ways of combining the drivers, e.g. a Euclidean distance is the square root of the sum of squares of the distances in standard deviations). Then we find say the nearest 4% of observations, and split them into “winners” (performing well) and “losers” (performing poorly). Where there is a significant difference between “winners” and “losers” – and this observation resembles the “losers” – we have identified either:
- an additional key driver to match on (if it’s a driver outside management control) or
- an additional aspect to success to consider adding to the success metric (if it’s a result rather than a driver) or
- an action item for improvement (if it’s a driver under management influence).
The look-alike methodology has several advantages over other approaches:
- Unlike a pure modelling approach, you are comparing against real observations rather than a synthetic fit across the whole database.This makes it a lot easier to cope with non-linearities, discontinuities, and local peculiarities.
- Unlike a “league tables” approach, the manager cannot say “but my business is different”.You have taken account of the differences that make a difference.
- It gets a reasonable sized comparison sample even if the database has relatively few observations, say 50 or more.
- If there are natural clusters it will find them and use them: if observations fall along a continuum that’s fine too.
- It considers many dimensions at once: if either the goalpost for success or the recipe for success requires getting several things right simultaneously, it will identify that more readily than many modelling approaches.
PIMS has wide practical experience of developing look-alikes in different situations; from cross-industry strategic business units (matching on competitive strength, market attractiveness, and capital/labour intensity) to various types of production plants or warehouses (matching on scale, complexity, product mix, automation and labour cost environment). We have developed different distance metrics (e.g. non-Euclidean and asymmetric), different ways of dealing with cross-sectional versus time-series comparisons, and different ways of showing results for different situations. If you want to compare against look-alikes – without being odious – talk to us first!